The Predictive Analytics & Inference Lab is a specialized research facility focused on advancing the field of predictive analytics and statistical inference. Our lab combines statistical modeling, data analysis techniques, and machine learning algorithms to extract valuable insights from data, make accurate predictions, and draw meaningful inferences. We aim to develop innovative methodologies, models, and tools to enable data-driven decision-making and solve complex problems in diverse domains.
Key Features of the Predictive Analytics & Inference Lab:
-
Predictive Modeling
We specialize in developing predictive models that leverage historical data to make accurate predictions about future events or outcomes. Our research focuses on regression analysis, time series forecasting, classification, and ensemble techniques to create robust predictive models
-
Statistical Inference
The lab emphasizes statistical inference to draw meaningful conclusions from data and estimate population parameters. We explore methods such as hypothesis testing, confidence intervals, and sampling techniques to provide reliable and interpretable results.
-
Data Mining and Pattern Recognition
We employ data mining techniques to discover patterns, associations, and relationships within datasets. Our lab applies methods such as clustering, association rule mining, and anomaly detection to uncover valuable insights and hidden patterns in complex data.
-
Feature Selection and Dimensionality Reduction
Our lab focuses on developing techniques to identify relevant features and reduce the dimensionality of data. By selecting informative features and reducing the complexity of datasets, we enhance the performance and interpretability of predictive models.
-
Optimization and Decision Analysis
We explore optimization techniques and decision analysis methodologies to support optimal decision-making processes. This includes techniques such as linear and integer programming, multi-criteria decision analysis, and decision trees to assist in complex decision scenarios.
-
Bayesian Inference
The lab employs Bayesian inference methods to incorporate prior knowledge and update beliefs based on observed data. We explore Bayesian networks, hierarchical models, and Markov Chain Monte Carlo (MCMC) methods to perform probabilistic inference and uncertainty quantification.
-
Causal Inference
We specialize in causal inference techniques to understand the cause-effect relationships between variables. Our lab employs methods such as randomized controlled trials, propensity score matching, and instrumental variable analysis to estimate causal effects and make causal claims.
-
Model Validation and Evaluation
The lab focuses on developing methodologies to validate and evaluate predictive models. We explore techniques such as cross-validation, model performance metrics, and calibration methods to ensure the reliability and generalizability of our models.
-
Real-World Applications
We apply predictive analytics and statistical inference techniques to various domains, including finance, marketing, healthcare, supply chain management, and social sciences. By addressing domain-specific challenges, we provide actionable insights and support evidence-based decision-making.
-
Collaboration and Industry Partnerships
The Predictive Analytics & Inference Lab actively collaborates with industry partners, research institutions, and experts. We work together on research projects, share knowledge, and engage in technology transfer to ensure our research outcomes have practical applications and real-world impact.
Through our research, innovation, and collaborations, the Predictive Analytics & Inference Lab aims to advance the field of predictive analytics and statistical inference, develop state-of-the-art methodologies and models, and contribute to data-driven decision-making and problem-solving in various domains.
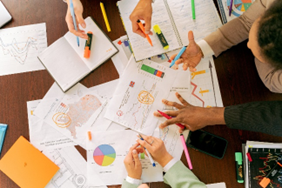